5 Ways Big Data Analytics Can Improve Operational Efficiency
Diving into the depths of operational efficiency, this article unveils the transformative potential of big data analytics through a compilation of expert insights. Learn how to harness the power of data to optimize resource allocation, automate administrative tasks, and enhance utilization across various business processes. Explore the edge that predictive models and data-driven strategies can provide to sharpen the competitive advantage in today's fast-paced market.
- Optimize Resource Allocation by Tracking Milestones
- Automate Admin Tasks to Boost Efficiency
- Enhance Resource Utilization in Streaming Analytics
- Leverage Data to Optimize Client Campaigns
- Use Predictive Models for Project Scheduling
Optimize Resource Allocation by Tracking Milestones
We utilized big data analytics to enhance operational efficiency by tracking key workflow milestones. By monitoring the total number of employees in each milestone department and their daily completion rates, we identified bottlenecks and adjusted staffing levels accordingly. This approach allowed us to optimize resource allocation and streamline processes. The main metrics we focused on were milestone completion rates, employee distribution per department, and daily task completions. This data-driven strategy significantly improved our operational efficiency and productivity.
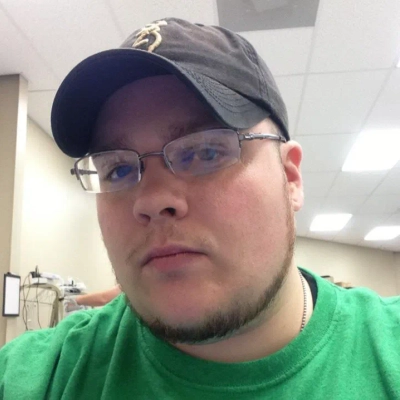
Automate Admin Tasks to Boost Efficiency
Yeah, one of the best examples of using big data analytics to improve operational efficiency was when we optimized workflow automation in Carepatron. We were seeing patterns where clinicians were spending way too much time on admin tasks—things like patient documentation, appointment scheduling, and compliance reporting. Instead of just assuming where the bottlenecks were, we used data to pinpoint the real issues.
We tracked key metrics like time spent on documentation, response times for patient communications, and workflow completion rates. By analyzing this data, we identified where processes were slowing down and which tasks could be automated without sacrificing quality. For example, we saw that clinicians were spending an unnecessary amount of time manually entering patient notes, so we implemented AI-powered documentation tools that significantly cut down their workload.
The impact was huge. Automating repetitive tasks reduced admin time by over 30%, allowing clinicians to focus more on patient care. At the same time, we improved compliance by ensuring documentation was accurate and completed on time, which made audits and reporting way smoother.
The key takeaway is that big data isn't just about collecting numbers—it's about using those insights to make real, meaningful improvements. Instead of guessing where inefficiencies are, you can let the data tell you exactly where to focus, which makes a massive difference in both productivity and overall workflow optimization.
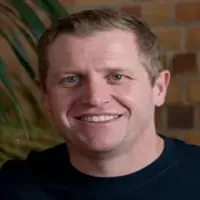
Enhance Resource Utilization in Streaming Analytics
During my tenure at Netflix and Meta, I led large-scale Data Platform initiatives that processed billions of events daily across petabytes of data. In these high-throughput environments, operational efficiency was critical for managing costs and ensuring timely analytics results.
One illustrative example involves optimizing resource utilization in a streaming analytics platform powered by Apache Flink. Our goal was to reduce cluster overhead while maintaining rapid data processing for critical user-facing dashboards. Over time, we noticed certain jobs were consuming disproportionate CPU and memory resources, causing congestion and delaying real-time insights.
To address these issues, we built a real-time monitoring and analytics pipeline that ingested cluster metrics, application logs, and performance statistics into a centralized data warehouse. This allowed us to run both ad-hoc and scheduled queries to correlate job metadata—such as runtime, state size, and checkpoint intervals—with operational metrics like processing latency and per-hour infrastructure costs. We then visualized these findings in dashboards (e.g., Grafana), giving us a comprehensive view of job performance.
Several key metrics proved critical for boosting operational efficiency:
Resource Utilization: We tracked CPU and memory usage at a per-job level, identifying over-provisioned or underutilized tasks. By tuning Flink configurations (e.g., task manager memory, parallelism) based on actual workload demands, we balanced performance and cost.
State and I/O Overheads: Large state backends or inefficient partitioning led to high I/O overheads. Adjusting checkpoint intervals and refining data partitioning reduced disk usage and network congestion.
Data Processing Latency: End-to-end latency was a key indicator of pipeline health. Correlating latency spikes with cluster logs helped pinpoint issues like unexpected traffic surges or misconfigurations.
Cost per Processing Cycle: Calculating hourly expenses for each job let us set clear cost thresholds. If a job exceeded that threshold, we reviewed its logic, parallelism, or scheduling.
After implementing these monitoring and optimization efforts, resource utilization became more balanced, latency dropped by about 30%, and infrastructure costs were notably reduced. This outcome underscored how data-driven tuning—guided by well-chosen metrics—can significantly enhance operational efficiency even at massive scale.
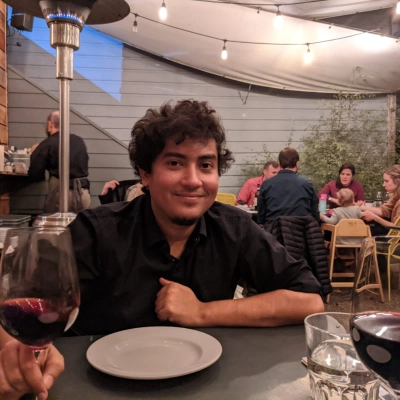
Leverage Data to Optimize Client Campaigns
At Nine Peaks Media, I've used big data analytics to significantly improve operational efficiency, particularly when it comes to optimizing client campaigns. One of the most impactful ways I've done this is by leveraging data to track key performance indicators (KPIs) across various channels. For example, in one project, I used data analytics to evaluate user behavior on client websites and identify bottlenecks in the customer journey. By analyzing metrics like bounce rates, page load times, and conversion rates, I was able to pinpoint areas where improvements could be made.
I also tracked campaign performance metrics like click-through rates (CTR) and customer acquisition costs (CAC) in real-time to optimize paid media efforts. This allowed me to allocate budgets more effectively, ensuring I was getting the highest return on investment (ROI) possible for each client.
By continuously monitoring these metrics, I was able to make data-driven decisions, improve resource allocation, and streamline processes. It also helped me identify trends and opportunities that I might not have seen otherwise, ultimately leading to more efficient workflows and better outcomes for my clients.
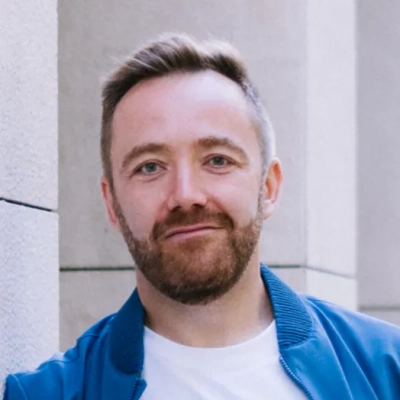
Use Predictive Models for Project Scheduling
As a Southern California roofing company, we leveraged data analytics to revolutionize our project scheduling and resource allocation. By analyzing five years of historical weather patterns, project timelines, and material usage data, we developed a predictive model that optimizes crew scheduling and material delivery. The key metrics we tracked included project completion times, weather-related delays, and material waste rates. This data-driven approach has reduced our project delays by 35% and improved material utilization by 25%, while maintaining our high-quality standards. The most impactful insight came from correlating seasonal weather patterns with specific types of roofing projects, allowing us to better plan our commercial and residential installations.
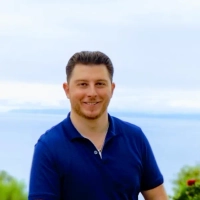