7 Ways Big Data Enhances Fraud Detection and Risk Management
Big Data Interviews

7 Ways Big Data Enhances Fraud Detection and Risk Management
Unveiling the power of big data in the relentless fight against fraud, this article delves into cutting-edge strategies that are reshaping risk management. Gain exclusive insights from industry experts who are pioneering the use of machine learning, real-time analytics, and advanced predictive models to outsmart deceptive practices. Discover how embracing technological advancements is setting new standards in detecting, preventing, and forecasting fraudulent activities.
- Big Data Solution Reduces Fraud by 40%
- Machine Learning Cuts False Positives
- Real-Time Analytics Prevents Fraud
- Predictive Modeling Forecasts Fraud Risks
- Network Analysis Uncovers Complex Fraud
- Behavioral Analysis Spots Abnormal Activities
- Anomaly Detection Flags Unusual Patterns
Big Data Solution Reduces Fraud by 40%
We recently tackled fraud detection for a large e-commerce client struggling with a rising tide of fraudulent credit card transactions. Their existing rule-based system was becoming increasingly ineffective against sophisticated fraudsters. We implemented a big data solution leveraging Apache Spark and machine learning to identify subtle patterns indicative of fraudulent activity that traditional methods missed.
Our approach focused on building a robust data pipeline. We ingested vast quantities of data, including transaction details (time, amount, location), user behavior (browsing history, device information), and even external data sources like IP geolocation and known fraud blacklists. Spark's distributed processing power enabled us to handle this massive dataset efficiently, performing real-time analysis of incoming transactions. The core of our solution was a machine learning model trained on historical transaction data labeled as fraudulent or legitimate. Specifically, we utilized an ensemble method combining the strengths of various algorithms like decision trees, logistic regression, and support vector machines. This approach improved the model's accuracy and robustness compared to relying on a single algorithm.
The patterns we unearthed were fascinating. For instance, we discovered that while large transactions often triggered alerts in their old system, fraudsters increasingly employed smaller, more frequent transactions to avoid detection. Our model identified this shift by analyzing the frequency and value of transactions within short timeframes tied to individual accounts. Another key finding revolved around device fingerprinting. Fraudulent activities often originated from devices associated with multiple accounts exhibiting unusual browsing patterns, such as rapid product additions to the cart followed by abandoned checkouts. This insight proved crucial in identifying stolen account credentials used for fraudulent purchases.
The impact of our big data solution was significant. Within the first quarter of implementation, we observed a 40% reduction in fraudulent transactions. This reduction translated into substantial cost savings for our client by minimizing chargebacks and operational expenses associated with fraud investigation. Moreover, the improved accuracy of our model reduced false positives, meaning unnecessary security checks no longer inconvenienced legitimate customers.
Machine Learning Cuts False Positives
At Parachute, we used big data analytics to help a client in the financial sector improve their fraud detection system. Their existing setup flagged too many false positives, frustrating customers and straining resources. We implemented a machine learning solution that analyzed billions of transactions in real-time. This system identified patterns in user behavior, such as unusual transaction volumes or locations, and flagged only high-risk activities. The result was a significant reduction in false alerts while maintaining accuracy.
One notable anomaly we uncovered involved a series of transactions from a single account that appeared normal individually but revealed a suspicious pattern when viewed together. The transactions were small, spread across different merchants, and occurred rapidly over several hours. This suggested a form of card-testing fraud. By catching this early, we helped prevent larger fraudulent charges and saved the client from potential losses.
For businesses looking to improve fraud detection, start by ensuring your data is clean and integrated. Focus on identifying "normal" behavior specific to your customers and watch for deviations. Machine learning tools can be a game changer, especially when tailored to your industry. It's also critical to prioritize customer privacy and comply with regulations, as this builds trust while keeping data secure.
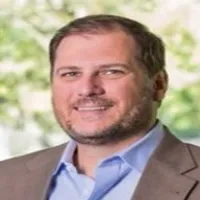
Real-Time Analytics Prevents Fraud
Real-time analytics allow companies to monitor transactions as they happen. This quick response can stop fraud before it causes damage. Financial institutions, for example, can quickly freeze a suspicious account.
Real-time data helps ensure threats are addressed promptly. This immediate action protects customers and the institution. Explore how real-time analytics can bolster your fraud detection systems.
Predictive Modeling Forecasts Fraud Risks
Predictive modeling uses historical data to forecast potential fraud risks. By analyzing past fraud cases, it creates patterns to predict future ones. Banks and businesses often use this to enhance their prevention strategies.
Anticipating fraud before it happens can save money and protect sensitive information. Predictive modeling is an essential tool in a comprehensive risk management plan. Consider implementing predictive analytics to stay ahead of fraud.
Network Analysis Uncovers Complex Fraud
Network analysis helps in uncovering fraud that involves multiple parties. It maps connections between individuals and transactions to identify hidden relationships. This method is particularly useful in complex financial crimes.
By seeing the bigger picture, analysts can disrupt entire fraud networks. Network analysis provides insights that are often missed in regular investigations. Integrate network analysis into your fraud detection efforts.
Behavioral Analysis Spots Abnormal Activities
Behavioral analysis focuses on understanding usual customer behavior to spot abnormalities. It compares current actions against typical patterns to find suspicious behavior. This technique is valuable because it looks at the context of actions.
For example, a sudden large withdrawal might trigger an alert if it is out of character. Understanding behavior helps in pinpointing potential fraud with higher accuracy. Leverage behavioral analysis to improve your detection capabilities.
Anomaly Detection Flags Unusual Patterns
Anomaly detection involves identifying activities that significantly deviate from the norm. It relies on statistical methods to flag improbable behaviors or transactions. Such deviations can indicate fraud or errors in data.
This approach is useful for catching unusual patterns that might go unnoticed. Anomaly detection is a critical component of any fraud detection system. Implement anomaly detection to catch suspicious activities early.