Emerging Trends in Big Data Analytics to Watch
Big Data Interviews

Emerging Trends in Big Data Analytics to Watch
Dive into the future of big data with emerging trends that are shaping the industry. This article uncovers the latest breakthroughs, featuring insights from renowned experts who are at the forefront of technological innovation. Explore how these advancements are revolutionizing the way data is analyzed and utilized, transforming businesses and industries worldwide.
- AI-Driven Analytics With Vector Databases
- Generative AI Models Answer 'Why' Questions
- Natural Language Chat Interfaces Revolutionize Building
- Edge Computing Enables Real-Time Decision-Making
- Real-Time Data Processing Transforms Industries
AI-Driven Analytics With Vector Databases
Real-time AI-driven analytics with vector databases is one new big data analytics trend that I find exciting because it is completely changing how companies derive insights from high-dimensional data. In contrast to conventional relational databases, vector databases such as FAISS, Pinecone, or Milvus use Approximate Nearest Neighbor (ANN) search on high-dimensional embeddings produced by deep learning models to facilitate effective similarity search on unstructured data (such as text, audio, and images). By making AI applications like anomaly detection, personalized search, and real-time recommendation systems possible, this trend is influencing the future. This trend is closely tied to Big Data since it addresses the issues of scalability, speed, and complexity in analyzing large amounts of unstructured and high-dimensional data.
Think of an e-commerce site whose recommendation engine is powered by vector databases. It employs a transformer-based paradigm (e.g., BERT for text or CLIP for images) to transform user interactions, product descriptions, and photos into embeddings rather than depending on simple collaborative filtering. FAISS is used to store and query these embeddings in real time, enabling the system to rapidly suggest products that are both visually and semantically comparable. This shows how AI-driven real-time insights will become the standard in Big Data analytics in the future by increasing user engagement and recommendation relevance.
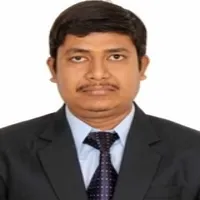
Generative AI Models Answer 'Why' Questions
Retail and e-commerce operations rely heavily on solutions such as demand forecasting, recommendation systems, inventory planning & optimization, pricing & markdown optimization, etc., for their day-to-day operations and planning. These solutions are either developed by internal data science teams or obtained through third-party SaaS systems. Either way, the main challenge the data science teams face is to answer the "why" questions based on the output of these algorithms. Business managers and operations planners need to understand why an algorithm generated a particular solution in order to trust the system. These are very difficult questions for non-domain experts to answer. Therefore, data scientists need to spend significant time backtracking the steps to determine what changes in the data impacted the final results. This approach is not scalable on a daily basis, and it also directly hampers productivity towards building new algorithms and solutions. In addition, a more significant business impact is that by the time the explanation is provided back to the users, the business/operational scenario is no longer relevant to implement the same solution. Training Generative AI models and developing various relevant AI Agents using big data provides a feasible pathway to crack this problem by appropriately integrating LLMs with the codebase of any SaaS solution through proper APIs developed by domain experts. Business users can ask their "why" questions through a natural-language interface and get meaningful and understandable answers. They can also do "what-if" scenario analysis through the same framework.
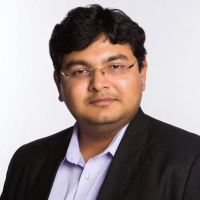
Natural Language Chat Interfaces Revolutionize Building
Natural language chat interfaces!
So much time is spent building. Building reports, building logic, building dashboards, etc.
AI can do the building, so we can focus on asking the right questions about our data.
There will be a shift from the "80% of the time is spent on cleaning up the data." More time for uncovering insights and resulting in more experimentation.
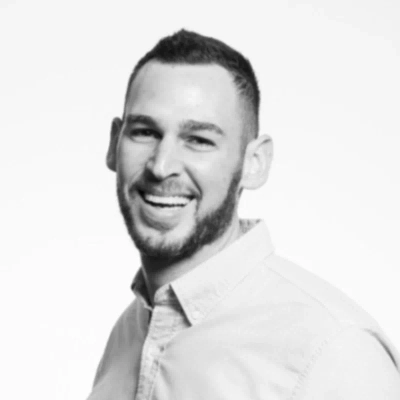
Edge Computing Enables Real-Time Decision-Making
One emerging trend in big data analytics that excites me is the rise of edge computing. This involves processing data closer to the source of generation, rather than sending it all to centralized cloud servers. With the increasing number of Internet of Things (IoT) devices and the need for real-time analysis, edge computing allows for faster insights, reduced latency, and more efficient bandwidth usage.
I see this trend shaping the future of data analysis by enabling real-time decision-making across industries such as health care, manufacturing, and autonomous vehicles. For example, in health care, edge computing can allow for the analysis of patient data in real time through wearable devices, enabling immediate responses to critical conditions. In manufacturing, it can optimize production lines by analyzing sensor data on the spot to prevent equipment failures before they happen.
By decentralizing the processing of data, edge computing is making data analysis faster, more efficient, and more responsive, paving the way for smarter, more agile systems that can make decisions in real time. This trend is likely to continue growing, especially as AI and machine learning models are integrated into edge devices, making them even more powerful and insightful.
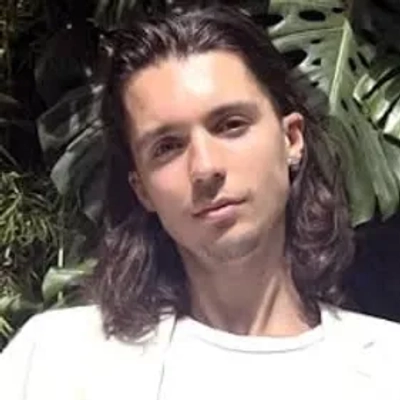
Real-Time Data Processing Transforms Industries
One emerging trend in big data analytics that excites me is real-time data processing. The days of waiting for weekly or monthly reports are behind us. Businesses are moving toward instant insights, allowing them to react faster and make smarter decisions. I've seen companies struggle with delayed data, making decisions based on outdated information. Now, with advancements like Snowpipe streaming and Dataflow Prime, organizations can act in real time, which is a game-changer. Real-time analytics is already transforming industries. In finance, milliseconds can mean millions of dollars. In retail, instant data helps adjust pricing, stock levels, and promotions on the fly. Walmart's hybrid cloud system, for example, enables real-time supply chain management, ensuring shelves stay stocked and customers find what they need. I've worked with businesses where even a few hours of delay in data analysis caused inefficiencies. With streaming data, those delays disappear. Businesses must be ready to manage and trust real-time insights. Faster data processing means a higher risk of acting on incomplete or inaccurate information. Data observability tools help ensure accuracy, but companies must invest in monitoring and governance. The goal isn't just speed—it's making sure decisions are based on fresh, reliable data. Those who embrace real-time analytics will have a competitive edge, responding faster and staying ahead in their industries.
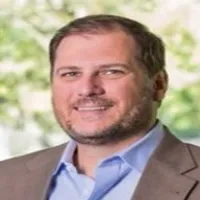