How Can Big Data Solutions Be Optimized for Better Performance?
Big Data Interviews
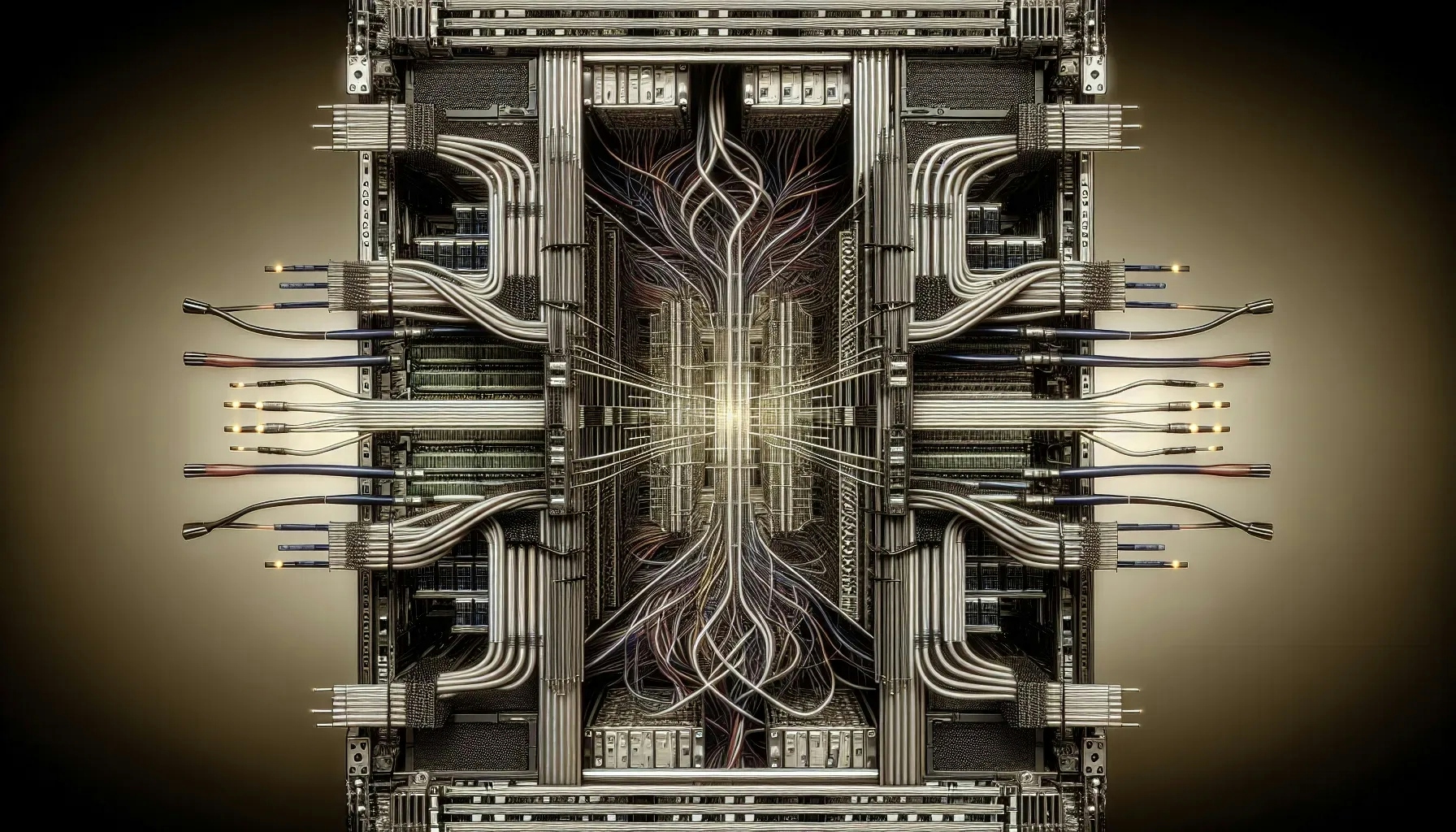
How Can Big Data Solutions Be Optimized for Better Performance?
From the strategic perspective of a Big Data Architect optimizing AdTech data processing, to the integration of advanced compression and deduplication techniques, we've compiled a series of success stories in big data optimization. Alongside expert insights, we also include additional answers that provide a broader spectrum of strategies used to enhance big data systems. These narratives not only highlight the technical improvements but also the impactful outcomes that followed.
- Optimized AdTech Data Processing
- Transition to Scalable Cloud Solutions
- Implement Parallel and In-Memory Processing
- Enhance with Machine Learning Predictions
- Prioritize Real-Time Data Analytics
- Integrate Compression and Deduplication Techniques
Optimized AdTech Data Processing
In the demanding landscape of adtech, where we handle a relentless influx of 500,000 queries per second (QPS) daily, processing and storing this data in near-real time for crucial business decisions presents a formidable challenge. Initially, we grappled with resource-intensive data fetching, utilizing a configuration of 20 executors, each boasting 8 cores and 8 GB of RAM. Recognizing the strain on resources, we embarked on a comprehensive analysis of our pipeline, leading to optimization measures that drastically enhanced efficiency. Through strategic adjustments, we transformed our setup to utilize 20 executors with reduced specifications of 2 cores and 4 GB of RAM each. Remarkably, this optimization not only met our processing needs but also enabled us to handle three times the data volume as before, ensuring our ability to make timely and informed decisions in the dynamic adtech industry.
Transition to Scalable Cloud Solutions
To optimize big data solutions, it's crucial to implement scalable and cloud-native data architectures. This involves using systems designed to expand rapidly to handle increasing data volumes without compromising performance. By migrating to a cloud-native platform, organizations can benefit from the flexibility and scalability provided by cloud services.
This adaptability ensures that data management systems can grow with the company's needs, allowing for the efficient processing of large quantities of information. Consider transitioning to a scalable, cloud-native data solution to enhance your data management capabilities.
Implement Parallel and In-Memory Processing
Utilizing parallel processing and in-memory computation can significantly improve the performance of big data solutions. Parallel processing divides large data sets into smaller chunks that can be processed concurrently, speeding up analysis and response times. On the other hand, in-memory computation stores data in RAM instead of slower disk drives, leading to faster access and processing speeds.
These approaches can drastically reduce the time required for data-intensive operations. Explore the potential for parallel processing and in-memory computation in your organization's data strategy for better performance.
Enhance with Machine Learning Predictions
Applying machine learning for predictive data analysis can lead to more optimized big data solutions. Machine learning algorithms can analyze large data sets to identify patterns and predict future trends, enhancing decision-making processes. Moreover, these predictive models can be refined over time to become more accurate and efficient.
As a result, organizations can proactively address potential issues and capitalize on opportunities. Integrate machine learning into your data analysis to uncover valuable insights and drive performance improvements.
Prioritize Real-Time Data Analytics
Optimization of big data solutions can also be realized through real-time processing and analytics. Real-time analytics provide instant insights by analyzing data as it is generated, enabling quicker decision-making and response to changing conditions. This immediacy can be a game-changer for businesses that require instantaneous data analysis to stay competitive or manage operations efficiently.
By prioritizing real-time data processing capabilities, organizations can ensure they are making informed decisions based on the most up-to-date information. Seek out technologies that enable real-time processing to remain agile in a fast-paced data environment.
Integrate Compression and Deduplication Techniques
Finally, leveraging data compression and deduplication techniques can refine the performance of big data solutions. Data compression reduces the size of data files, which can expedite transfer and storage processes, while deduplication removes duplicate copies of repeating data, enhancing storage efficiency. These methods not only save on storage costs but also improve the speed of data retrieval and processing.
Efficient data management practices such as these can lead to significant performance gains. Assess your data systems for opportunities to integrate compression and deduplication techniques.