Predicting Future Trends With Big Data: 4 Techniques
Unlock the potential of big data to anticipate the future with cutting-edge techniques. This article provides a deep dive into advanced forecasting models, backed by insights from top industry experts. Explore how predictive analytics are shaping decisions in B2B retail, cybersecurity, online content creation, and seasonal product demand.
- Developed Forecasting Model for B2B Retailer
- Predicted Phishing Attacks for Law Firm
- Predicted Video Trends Using YouTube Data
- Predicted Holiday Product Demand
Developed Forecasting Model for B2B Retailer
Retail operations and planning heavily rely on accurate demand forecasts. I was tasked with developing a forecasting model for delivery operations for one of the largest B2B retailers in the US. After the initial COVID-19 lockdowns, as vaccinations were administered, different counties and states in the US began gradually reopening in a controlled manner, leading to an increase in out-of-home activities. In some areas, a rise in infections again led to the re-imposition of partial lockdowns. The CDC and other governmental agencies maintained various big databases with the then-current and projected percentages of lockdowns for each county and state. This lockdown data was crucial for adjusting forecasts for the B2B retailer, where a significant portion of sales depends on people returning to their workplaces.
There have been other instances where external factors in the form of big data, such as extreme weather events or trends on social media, played a vital role in refining forecasts. The key point here is that the more future events differ from historical ones, the more essential it becomes to incorporate relevant external big data into generating accurate forecasts. If future events closely mirror those of the past, a forecasting system would be self-sufficient.
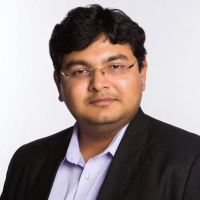
Predicted Phishing Attacks for Law Firm
At Tech Advisors, we help businesses stay ahead of cybersecurity threats by using predictive analytics. One instance where we applied big data to predict future trends was in detecting phishing attacks for a law firm client. Cybercriminals constantly refine their tactics, so we analyzed historical attack patterns, email metadata, and employee engagement with phishing simulations. Using classification models like logistic regression and decision trees, we identified high-risk users and patterns in fraudulent emails. This allowed us to develop targeted training and adaptive email filtering, reducing phishing-related incidents by over 60% in three months.
We also used time series models to anticipate peak phishing attempts. Attackers often strike during specific business cycles--end of the quarter, tax season, or before long weekends. We implemented an ARIMA model to assess past attack frequencies and predict when the next surge might occur. With this data, we advised the firm to schedule extra security awareness training and reinforce authentication measures ahead of high-risk periods. As a result, the firm saw a measurable drop in successful phishing attempts, improving both security and employee confidence in handling suspicious emails.
For any business dealing with cybersecurity threats, predictive analytics is a powerful tool. It's important to collect quality data and choose the right models to extract useful insights. In our case, blending classification and time series models gave us a clear picture of risk patterns. Any company handling sensitive data should consider similar predictive approaches to mitigate threats before they become crises.
Predicted Video Trends Using YouTube Data
I used big data analysis on YouTube performance metrics to predict which video topics would gain traction before they peaked. By analyzing watch time, audience retention, and search trends, I identified emerging patterns in B2B video marketing--specifically, the growing demand for AI-powered video editing tools.
I employed Google Trends, YouTube Analytics, and keyword research tools to track rising search interest and engagement shifts. Based on this data, I created content around AI-driven video strategies before the trend exploded. The prediction was spot-on--those videos quickly outperformed previous uploads, driving higher watch times and 3x more engagement than standard industry topics. This data-driven approach ensured I stayed ahead of the curve, rather than reacting after the trend was saturated.
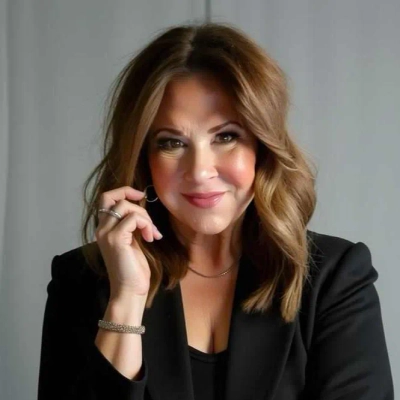
Predicted Holiday Product Demand
I worked on product based project for a short time(during the holiday season). In that, I used historical sales data to predict product demand during the holiday season.
I gathered data from past years and studied it to spot patterns in customer buying habits. I checked which products were most popular, when sales peaked the most, and how things like promotions or events affected what people bought. I applied regression analysis to understand how demand changed throughout the year. Then, I used time series forecasting to predict future sales.
We made predictions that were 80-85% accurate. This helped the business stock the right products and avoid running out of inventory. It also made it easier to run successful marketing campaigns for popular items.
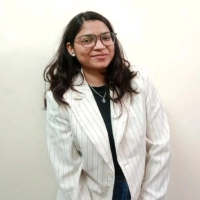