What Are Innovative Ways Machine Learning Extracts Insights from Big Data?
Big Data Interviews

What Are Innovative Ways Machine Learning Extracts Insights from Big Data?
Harnessing the power of machine learning to unlock the full potential of big data, we've gathered insights from an Expert Data Scientist on implementing predictive maintenance. Alongside industry leaders, we also present additional answers that span the gamut from real-time data stream processing to advancing predictive analytics with deep learning. These innovative approaches reveal the transformative impact machine learning has on extracting meaningful insights from vast datasets.
- Implement Predictive Maintenance
- Visualize Data with PCA
- Enhance E-Commerce Recommendations
- Automate Anomaly Detection
- Process Data Streams in Real Time
- Extract Insights with NLP
- Optimize with Reinforcement Learning
- Advance Predictive Analytics with Deep Learning
Implement Predictive Maintenance
Predictive maintenance is one creative application of machine learning that I have experienced, which concludes a large volume of data with variety. This method reduces downtime and maximizes maintenance schedules by utilizing past data from sensors and machinery to forecast when equipment is likely to break or need maintenance.
Operational Steps:
Data collection: Sensors are affixed to machinery to gather diverse forms of data, including vibration, temperature, pressure, and more. Usually, this data is gathered on a regular basis.
Feature engineering: It involves the analysis of raw sensor data by engineers and data scientists to find pertinent traits that may signal the machinery's health. These characteristics might be variations in temperature, pressure, or vibration frequency patterns.
Model Training: Using historical data (a large number of data points), machine learning algorithms, like supervised learning models such as neural networks, random forests, and gradient boosting machines, are trained. This information covers both typical operating conditions and incidents of maintenance or equipment failure.
Prediction: Using real-time sensor data, the trained model can forecast when machinery is likely to break down. By initiating maintenance prior to a malfunction, these forecasts help minimize downtime and avert expensive repairs.
Some major insights from the novel application of machine learning in predictive maintenance:
Optimized Maintenance Schedules: Conventional maintenance plans frequently depend on predetermined timeframes or reactive fixes for malfunctions. Organizations can customize maintenance schedules based on the actual state of the equipment thanks to machine learning, which maximizes resource efficiency and reduces unnecessary maintenance.
Data-Driven Insights: Detailed big data analysis and machine learning model building are necessary for the predictive maintenance process. Important information on the functionality and condition of the equipment is produced by this process, which can be utilized to guide initiatives for ongoing improvement and make informed decisions.
Organizations in a variety of industries stand to gain a great deal from the adoption of machine learning in predictive maintenance, including reduced costs, increased productivity, and useful insights from big data.
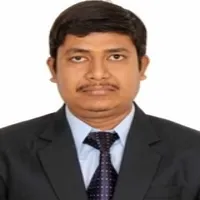
Visualize Data with PCA
I have experience in dealing with large amounts of unannotated images and videos collected from various real-world locations. The data we get is generally unstructured and in the magnitude of TBs.
Understanding the data by going through all the images is humanly not possible. In this situation, one of the approaches I use to understand it at a high level is calculating the embeddings using any feature extraction model and then performing PCA and plotting them on a 2D space. This gives a very clear insight into the whole data and its distribution. It also shows the outliers and similar-looking samples on a larger picture.
Enhance E-Commerce Recommendations
We had about 33.7 million rows of raw data from an e-commerce website (for a client, of course).
Machine learning was the only approach for this; our team of developers trained the model to analyze the data and work on cause and effect. Using machine learning, we were able to accurately implement the 'people also buy' section on the website.
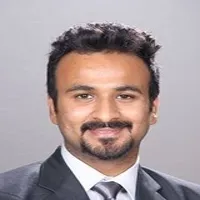
Automate Anomaly Detection
Automated anomaly detection systems leverage machine learning algorithms to monitor data streams vigilantly, spotting unusual patterns or irregularities that deviate from the norm. This type of system is invaluable for data engineers as it helps to flag potential issues in vast datasets swiftly, leading to quicker resolutions and maintaining data integrity. By identifying these outliers, businesses can prevent potential fraud, system failures, or operational bottlenecks before they escalate.
Such proactive monitoring can also save time and resources otherwise needed for manual data analysis. To enhance your data monitoring, start exploring automated anomaly detection tools today.
Process Data Streams in Real Time
Real-time stream processing is a groundbreaking method afforded by machine learning, where incoming data is immediately analyzed and processed. This technology enables data engineers to make instantaneous decisions based on current information, leading to more agile and responsive business strategies. It bypasses the traditional batch processing delays, facilitating real-time insights that can revolutionize customer experiences, operational efficiency, and market adaptability.
Such immediacy can be a game-changer in industries where time is of the essence. Consider incorporating real-time stream processing to accelerate your decision-making capabilities.
Extract Insights with NLP
Natural language processing (NLP), a facet of machine learning, is transforming the way data engineers can extract meaning and insights from text-heavy datasets. By analyzing and understanding human language, NLP can uncover trends and patterns in social media feeds, customer reviews, and support tickets. It opens up possibilities for improved sentiment analysis, more effective content recommendations, and better-targeted marketing campaigns.
By tapping into semantic analysis, organizations can connect more deeply with their clients' needs and preferences. Dive into the world of NLP to unlock the potential of your text data.
Optimize with Reinforcement Learning
Reinforcement learning, an area of machine learning, specializes in optimizing decision-making processes within data systems by using trial and error to discover efficient pathways. Data engineers can employ this technique to improve data workflows, automatically adjusting operations based on performance feedback. This leads to an increasingly proficient handling of routine tasks, freeing up valuable time for strategic analysis and innovation.
Moreover, this adaptive approach reduces error rates and boosts overall productivity. Explore how reinforcement learning can streamline your data operations for leaner, smarter workflow management.
Advance Predictive Analytics with Deep Learning
Deep learning, a complex branch of machine learning, is pushing the boundaries of predictive analytics. With its ability to digest and learn from large volumes of data, it supplies data engineers with the tools to foresee trends and user behaviors with a high degree of accuracy. This predictive power is enabling companies to get a step ahead in market forecasting, risk assessment, and strategic planning.
By forecasting future outcomes, businesses can craft preemptive measures to stay competitive. To stay ahead of the curve, start integrating deep learning techniques into your predictive analytics processes.