Why is Stream Processing Critical for Data Pipelines in Data Engineering?
When it comes to the pivotal role of stream processing in data pipelines, a Data Engineer reveals why they chose Apache Spark for real-time ETL. Alongside this expert perspective, we've gathered additional answers that highlight various critical instances and tools employed in the field. From boosting efficiency with event-driven processing to adapting infrastructure for high-throughput, discover the diverse experiences that underline the importance of stream processing.
- Choose Apache Spark for Real-Time ETL
- Leverage Real-Time Analytics for Decisions
- Accelerate Insights with Stream Processing
- Ensure Uninterrupted Data Flow
- Adapt Infrastructure for High-Throughput
- Boost Efficiency with Event-Driven Processing
Choose Apache Spark for Real-Time ETL
When you need to analyze data in real time to power analytical products or dashboards, it's mission-critical to use ETL so that the data is cleansed and processed.
Here, I would opt for Apache Spark, which is a popular tool for stream processing because it can handle large data volumes and distribute the processing across multiple computing instances for faster results.
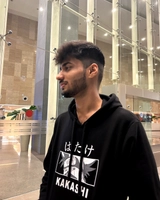
Leverage Real-Time Analytics for Decisions
Stream processing is a technique employed in managing data that is continuously generated by different sources. It's essential because it allows for the real-time analysis of data, leading to immediate insights that can help steer business decisions in the right direction. Whether it's monitoring user activity on a website or tracking live transaction data, stream processing enables businesses to react quickly to new information.
This capability of instant analytics is vital in an era where data is constantly flowing and immediate responses can provide competitive advantages. Discover how to leverage real-time analytics in your operations to make swift decisions.
Accelerate Insights with Stream Processing
In the realm of data engineering, reducing the time it takes to gain insights from data is like boosting the speed of an engine. Stream processing plays a pivotal role in accelerating this process by drastically cutting down latency. This allows data to move swiftly through the pipeline, making it ready for analysis much faster than traditional batch processing methods.
Quicker insights mean that businesses can respond to trends and anomalies in near real-time, which is crucial in a data-driven landscape where even the smallest delay can be costly. Explore stream processing to accelerate your insights and stay ahead of the curve.
Ensure Uninterrupted Data Flow
Stream processing is synonymous with a beating heart in the body of data engineering, continuously pumping data in and through the system. This non-stop flow is essential for applications that need immediate data, such as fraud detection systems or live financial tickers. By supporting ongoing data ingestion and processing, stream processing enables systems to maintain up-to-date information, which is fundamental for accuracy in many analytics applications.
It's a dynamic landscape where information never sleeps, and systems that support such robust data flows are at the forefront. Consider adopting a stream processing framework that allows uninterrupted data flow for your analytical needs.
Adapt Infrastructure for High-Throughput
The scalability and high-throughput capabilities of stream processing are indispensable in today's vast data ecology. By handling data in motion, it ensures that systems can manage large volumes of information swiftly and efficiently, thereby supporting the growth of the organization's data needs over time. Stream processing engines are adept at dealing with thousands to millions of events per second, making them an ideal choice for organizations that expect their data to scale.
Scalability also means that future expansions can be managed with ease, maintaining system performance under increased loads. Assess your system's scalability and adapt your infrastructure to be ready for high-throughput stream processing.
Boost Efficiency with Event-Driven Processing
For systems that operate based on events, such as notifications or real-time alerts, stream processing is the key to achieving peak efficiency. By immediately processing data as it arrives, these event-driven architectures can trigger instant responses, whether it’s in monitoring systems for unusual patterns or sending instant messages based on user activities. This boosts the overall efficiency, allowing businesses to operate more smoothly and effectively meet user demands.
Timeliness and responsiveness in processing events also help in keeping systems proactive rather than reactive. Improve your event-driven system by implementing stream processing for increased efficiency.